Klasifikasi Sinyal Elektro Encheplao Graph (EEG) menggunakan Jaringan Syaraf Tiruan Back Propagation Neural Network
Keywords:
Sinyal EEG, Elektroensefalografi, Klasifikasi sinyal otakAbstract
Judul : Klasifikasi Sinyal Elektro Encheplao Graph (EEG) menggunakan Jaringan Syaraf Tiruan Back Propagation Neural Network
- BAB I Pendahuluan
- BAB II Sinyal Elektro Enchepalo Graph (EEG)
- BAB III Jaringan syaraf tiruan Backpropagation
- BAB IV Ekstrasi Sinyal EEG Menggunakan Metode Fast Fourier Transform (FFT)
- BAB V Ekstrasi Sinyal EEG Menggunakan Metode Transformasi Wavelet
- BAB VI Ekstrasi Sinyal EEG Menggunakan Metode Regresi
Editor: M.Tanzil Multazam & Mahardika Darmawan Kusuma Wardana
Published by:
Universitas Muhammadiyah Sidoarjo Press, Sidoarjo, 2024
ISBN:
- ISBN
Deskripsi :
Penelitian ini berfokus pada klasifikasi sinyal EEG dengan memanfaatkan Jaringan Syaraf Tiruan (JST), khususnya metode Back Propagation Neural Network (BPNN). EEG merupakan sinyal listrik yang dihasilkan oleh aktivitas otak dan direkam melalui elektroda yang ditempatkan di kulit kepala. Analisis dan klasifikasi sinyal EEG menjadi penting dalam berbagai bidang seperti neurosains, medis (terutama dalam diagnosis epilepsi, gangguan tidur, dan brain-computer interface), serta penelitian kognitif.
Downloads
References
J. S. Kumar and P. Bhuvaneswari, “Analysis of electroencephalography (EEG) signals and its categorization - A study,” Procedia Eng., vol. 38, pp. 2525–2536, 2012, doi: 10.1016/j.proeng.2012.06.298.
M. Teplan and Institute, “Fundamentals of Eeg Measurement,” AAAI Fall Symp. - Tech. Rep., vol. FS-12-04, no. 2, pp. 59–64, 2012.
Z. A. A. Alyasseri, A. T. Khader, and M. A. Al-Betar, “Electroencephalogram signals denoising using various mother wavelet functions: A comparative analysis,” ACM Int. Conf. Proceeding Ser., vol. Part F131372, no. October, pp. 100–105, 2017, doi: 10.1145/3132300.3132313.
E. S. Yousif, A. S. Abdulbaqi, A. Z. Hameed, and M. N. Saif Al-Din, “Electroencephalogram Signals Classification Based on Feature Normalization,” IOP Conf. Ser. Mater. Sci. Eng., vol. 928, no. 3, 2020, doi: 10.1088/1757-899X/928/3/032028.
A. A. A. Meidiary, A. M. Gelgel, and I. G. N. P. Putra, “Electroencephalogram (EEG) features and clinical presentation in the elderly patient at neurologic policlinic Sanglah General Hospital between July 2015-2017 period,” Bali Med. J., vol. 8, no. 2, pp. 667–671, 2019, doi: 10.15562/bmj.v8i2.1484.
N. Disorders, “The Epilepsies and Seizures”.
E. Board, “Epilepsy : Report by the director - general,” Development, vol. 1, no. October, pp. 1–6, 2019, [Online]. Available: https://apps.who.int/iris/handle/10665/355987
P. Fulpatil and Y. Meshram, “Analysis of EEG Signals with the Effect of Meditation,” Int. J. Eng. Res.Appl. www.ijera.com, vol. 4, no. 6, pp. 51–53, 2014, [Online]. Available:www.ijera.com
M. M. Siddiqui, S. Rahman, S. H. Saeed, and A. Banodia, “EEG Signals Play Major Role to diagnose Sleep Disorder,” Int. J. Electron. Comput. Sci. Eng., vol. 503, no. June 2013, pp. 503–505, 1956.
M. M. Siddiqui, S. Rahman, S. H. Saeed, and A. Banodia, “EEG Signals Play Major Role to diagnose Sleep Disorder,” Int. J. Electron. Comput. Sci. Eng., vol. 503, pp. 503–505, 1956.
V. Pandey, A. Agarwal, and M. M. Siddiqui, “Sleep disorders and eeg recording,” no. November, pp.206–210, 1956.
V. Menon and S. Crottaz-Herbette, “Combined EEG and fMRI Studies of Human Brain Function,” Int. Rev. Neurobiol., vol. 66, no. 05, pp. 291–321, 2005, doi: 10.1016/S0074-7742(05)66010-2.
A. Melnik, W. D. Hairston, D. P. Ferris, and P. König, “EEG correlates of sensorimotor processing: Independent components involved in sensory and motor processing,” Sci. Rep., vol. 7, no. 1, 2017, doi: 10.1038/s41598-017-04757-8.
A. Turnip et al., “Detection of Drug Effects on Brain Activity using EEG-P300 with Similar Stimuli,” IOP Conf. Ser. Mater. Sci. Eng., vol. 220, no. 1, 2017, doi: 10.1088/1757-899X/220/1/012042.
K. L. Son et al., “Neurophysiological features of Internet gaming disorder and alcohol use disorder: A resting-state EEG study,” Transl. Psychiatry, vol. 5, no. July, pp. 2–7, 2015, doi: 10.1038/tp.2015.124.
M. Balconi, S. Campanella, and R. Finocchiaro, “Web addiction in the brain: Cortical oscillations, autonomic activity, and behavioral measures,” J. Behav. Addict., vol. 6, no. 3, pp. 334–344, 2017, doi:10.1556/2006.6.2017.041.
I. Ahmad et al., “EEG-Based Epileptic Seizure Detection via Machine/Deep Learning Approaches: A Systematic Review,” Comput. Intell. Neurosci., vol. 2022, 2022, doi: 10.1155/2022/6486570.
A. Eviyanti, H. Hindarto, Sumarno, and H. A. A. Duddin, “Epilepsi detection system based on EEG record using neural network backpropagation method,” J. Phys. Conf. Ser., vol. 1381, no. 1, 2019, doi:10.1088/1742-6596/1381/1/012037.
S. Nefti and J. O. Gray, “Advances in cognitive systems,” Adv. Cogn. Syst., no. January, pp. 1–503,2010, doi: 10.1049/PBCE071E.
R. Thenius, P. Zahadat, and T. Schmickl, “EMANN - a model of emotions in an artificial neural network,” no. September 2013, pp. 830–837, 2013, doi: 10.7551/978-0-262-31709-2 ch122.
C. K. Behera, T. K. Reddy, L. Behera, and B. Bhattacarya, “Artificial neural network based arousal detection from sleep electroencephalogram data,” I4CT 2014 - 1st Int. Conf. Comput. Commun. Control Technol. Proc., no. September, pp. 458–462, 2014, doi: 10.1109/I4CT.2014.6914226.
M. Ronzhina, O. Janoušek, J. Kolářová, M. Nováková, P. Honzík, and I. Provazník, “Sleep scoring using artificial neural networks,” Sleep Med. Rev., vol. 16, no. 3, pp. 251–263, 2012, Bab 2 Judul Bab 39 doi:10.1016/j.smrv.2011.06.003.
J. Barragán, E. F. Estrada, P. A. Nava, and H. Nazeran, “EEG-based Classification of Sleep Stages using Artificial Neural Networks,” 5th Int. Work. Biomed. Signal Interpret., pp. 0–3, 2005.
A. Subasi, M. K. Kiymik, A. Alkan, and E. Koklukaya, “Neural network classification of EEG signals by using AR with MLE preprocessing for epileptic seizure detection,” Math. Comput. Appl., vol. 10, no. 1, pp. 57–70, 2005, doi: 10.3390/mca10010057.
V. T. N. Nguyen, V. T. Huynh, and T. H. H. Nguyen, “Electroencephalography analysis using neural network,” NICS 2018 - Proc. 2018 5th NAFOSTED Conf. Inf. Comput. Sci., no. May 2019, pp.144–147, 2019, doi: 10.1109/NICS.2018.8606797.
I. Rakhmatulin, “Review of Neural Networks in the EEG Signal Recognition,” SSRN Electron. J., no. November, pp. 1–15, 2021, doi: 10.2139/ssrn.3765947.
L. T. Car, “Hans berger ( 1873-1941 ) - The history of electroencephalography,” no. February 2005, 2016.
M. Rosinova, M. Lojka, J. Stas, and J. Juhar, “Voice command recognition using EEG signals,” Proc. Elmar - Int. Symp. Electron. Mar., vol. 2017-September, no. September, pp. 153 156, 2017, doi: 10.23919/ELMAR.2017.8124457.
K. Mohammad and S. Hamo, “Emotion Recognition and Authentication Based on Electroencephalogram ( EEG ) Signals,” pp. 1–11, 1924.
C. Caloian, “Use of EEG Signal to Detect Brain Patterns Supervisor : Frank Rudzicz Related Work,” pp. 1–23, 2013.
U. M. D. E. C. D. E. Los, No 主観的健康感を中心とした在宅高齢者における 健康関連指標に関する共分散構造分析Title.
H. V. D. Parunak, S. H. Brooks, S. Brueckner, and R. Gupta, “Apoptotic stigmergic agents for real-time swarming simulation,” AAAI Fall Symp. - Tech. Rep., vol. FS-12-04, pp. 59–64, 2012.
M. Zimmerman, N. A. Peterson, and M. A. Zimmerman, “Beyond the Individual : Toward a Nomological Network of Organizational Empowerment Beyond the Individual : Toward a Nomological Network of Organizational Empowerment,” vol. 34, no. October 2004, 2016, doi: 10.1023/B.
S. Kotte and J. R. K. Kumar Dabbakuti, “Methods for removal of artifacts from EEG signal: A review,” J. Phys. Conf. Ser., vol. 1706, no. 1, 2020, doi: 10.1088/1742-6596/1706/1/012093.
J. J. Riviello, “Pediatric EEG abnormalities,” Clin. Neurophysiol. Prim., pp. 179–204, 2007, doi: 10.1007/978-1-59745-271-7_11.
A. K. Singh and S. Krishnan, “Trends in EEG signal feature extraction applications,” Front. Artif. Intell., vol. 5, 2023, doi: 10.3389/frai.2022.1072801.
K. A. I. Aboalayon, M. Faezipour, W. S. Almuhammadi, and S. Moslehpour, “Sleep stage classification using EEG signal analysis: A comprehensive survey and new investigation,” Entropy, vol. 18, no. 9, 2016, doi: 10.3390/e18090272.
I. H. Sarker, “Machine Learning: Algorithms, Real-World Applications and Research Directions,” SN Comput. Sci., vol. 2, no. 3, pp. 1–21, 2021, doi: 10.1007/s42979-021-00592-x.
V. Ranganathan and S. Natarajan, “A New Backpropagation Algorithm without Gradient Descent,” no. January, 2018, [Online]. Available: http://arxiv.org/abs/1802.00027
O. A. Montesinos López, A. Montesinos López, and J. Crossa, Multivariate Statistical Machine Learning Methods for Genomic Prediction. 2022. doi: 10.1007/978-3-030-89010-0.
A. Wanto et al., “Levenberg-Marquardt Algorithm Combined with Bipolar Sigmoid Function to Measure Open Unemployment Rate in Indonesia,” pp. 22–28, 2021, doi: 10.5220/0010037200220028.
S. Rodini, “Analytical derivatives of neural networks,” Comput. Phys. Commun., vol. 270, pp. 1–12, 2022, doi: 10.1016/j.cpc.2021.108169.
N. Benvenuto and F. Piazza, “On the Complex Backpropagation Algorithm,” IEEE Trans. Signal Process., vol. 40, no. 4, pp. 967–969, 1992, doi: 10.1109/78.127967.
P. Munro, “Backpropagation,” Encycl. Mach. Learn. Data Min., pp. 93–97, 2017, doi: 10.1007/978-1- 4899-7687-1_51.
P. Heckbert, “Fourier Transforms and the Fast Fourier Transform (FFT) Algorithm,” Notes Comput.Graph., vol. 3, no. 2, pp. 15–463, 1995.
H. Hindarto and S. Sumarno, “Feature Extraction of Electroencephalography Signals Using Fast Fourier Transform,” CommIT (Communication Inf. Technol. J., vol. 10, no. 2, p. 49, 2016
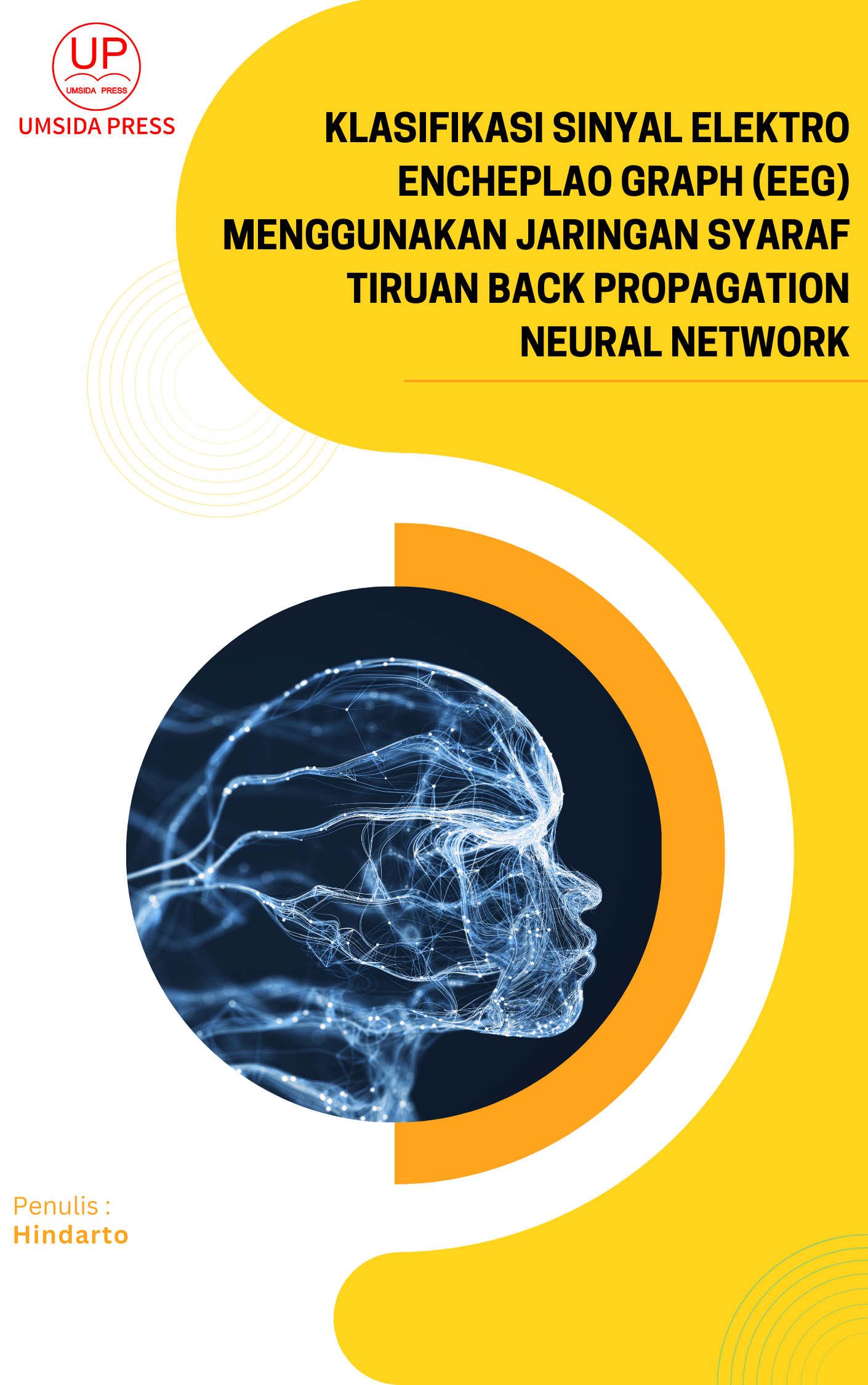
Published
How to Cite
License
Authors retain copyright and grant the Umsida Press right of first publication with the work simultaneously licensed under a Creative Commons Attribution 4.0 International License that allows others to share the work with an acknowledgement of the work's authorship and initial publication in this platform.